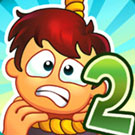
multidimensional wasserstein distance python
Figure 4. Given two empirical measures each with :math:`P_1` locations Wasserstein distance is often used to measure the difference between two images. . Making statements based on opinion; back them up with references or personal experience. can this be accelerated within the library? You can also look at my implementation of energy distance that is compatible with different input dimensions. If you liked my writing and want to support my content, I request you to subscribe to Medium through https://rahulbhadani.medium.com/membership. Wasserstein Distance) for these two grayscale (299x299) images/heatmaps: Right now, I am calculating the histogram/distribution of both images. What should I follow, if two altimeters show different altitudes? [Click on image for larger view.] weight. # scaling "decay" coefficient (.8 is pretty close to 1): # Number of samples, dimension of the ambient space, # Output one index per "line" (reduction over "j"). By clicking Accept all cookies, you agree Stack Exchange can store cookies on your device and disclose information in accordance with our Cookie Policy. The computed distance between the distributions. Please note that the implementation of this method is a bit different with scipy.stats.wasserstein_distance, and you may want to look into the definitions from the documentation or code before doing any comparison between the two for the 1D case! Sorry, I thought that I accepted it. One method of computing the Wasserstein distance between distributions , over some metric space ( X, d) is to minimize, over all distributions over X X with marginals , , the expected distance d ( x, y) where ( x, y) . wasserstein-distance GitHub Topics GitHub WassersteinEarth Mover's DistanceEMDWassersteinppp"qqqWasserstein2000IJCVThe Earth Mover's Distance as a Metric for Image Retrieval See the documentation. Calculate Earth Mover's Distance for two grayscale images that must be moved, multiplied by the distance it has to be moved. python - Intuition on Wasserstein Distance - Cross Validated It is denoted f#p(A) = p(f(A)) where A = (Y), is the -algebra (for simplicity, just consider that -algebra defines the notion of probability as we know it. L_2(p, q) = \int (p(x) - q(x))^2 \mathrm{d}x The Wasserstein distance between (P, Q1) = 1.00 and Wasserstein (P, Q2) = 2.00 -- which is reasonable. To subscribe to this RSS feed, copy and paste this URL into your RSS reader. Great, you're welcome. # The Sinkhorn algorithm takes as input three variables : # both marginals are fixed with equal weights, # To check if algorithm terminates because of threshold, "$M_{ij} = (-c_{ij} + u_i + v_j) / \epsilon$", "Barycenter subroutine, used by kinetic acceleration through extrapolation. Gromov-Wasserstein example. By clicking Post Your Answer, you agree to our terms of service, privacy policy and cookie policy. What should I follow, if two altimeters show different altitudes? Then we have: C1=[0, 1, 1, sqrt(2)], C2=[1, 0, sqrt(2), 1], C3=[1, \sqrt(2), 0, 1], C4=[\sqrt(2), 1, 1, 0] The cost matrix is then: C=[C1, C2, C3, C4]. To learn more, see our tips on writing great answers. Wasserstein in 1D is a special case of optimal transport. \[\alpha ~=~ \frac{1}{N}\sum_{i=1}^N \delta_{x_i}, ~~~ \beta ~=~ \frac{1}{M}\sum_{j=1}^M \delta_{y_j}.\]. Why does the narrative change back and forth between "Isabella" and "Mrs. John Knightley" to refer to Emma's sister? Wasserstein distance, total variation distance, KL-divergence, Rnyi divergence. The Jensen-Shannon distance between two probability vectors p and q is defined as, D ( p m) + D ( q m) 2. where m is the pointwise mean of p and q and D is the Kullback-Leibler divergence. @LVDW I updated the answer; you only need one matrix, but it's really big, so it's actually not really reasonable. Thanks for contributing an answer to Stack Overflow! In other words, what you want to do boils down to. # The y_j's are sampled non-uniformly on the unit sphere of R^4: # Compute the Wasserstein-2 distance between our samples, # with a small blur radius and a conservative value of the. Values observed in the (empirical) distribution. Browse other questions tagged, Where developers & technologists share private knowledge with coworkers, Reach developers & technologists worldwide. sig2): """ Returns the Wasserstein distance between two 2-Dimensional normal distributions """ t1 = np.linalg.norm(mu1 - mu2) #print t1 t1 = t1 ** 2.0 #print t1 t2 = np.trace(sig2) + np.trace(sig1) p1 = np.trace . When AI meets IP: Can artists sue AI imitators? To analyze and organize these data, it is important to define the notion of object or dataset similarity. # Author: Erwan Vautier <erwan.vautier@gmail.com> # Nicolas Courty <ncourty@irisa.fr> # # License: MIT License import scipy as sp import numpy as np import matplotlib.pylab as pl from mpl_toolkits.mplot3d import Axes3D . PhD, Electrical Engg. There are also "in-between" distances; for example, you could apply a Gaussian blur to the two images before computing similarities, which would correspond to estimating Python. Asking for help, clarification, or responding to other answers. How do you get the logical xor of two variables in Python? In Figure 2, we have two sets of chess. ", sinkhorn = SinkhornDistance(eps=0.1, max_iter=100) The 1D special case is much easier than implementing linear programming, which is the approach that must be followed for higher-dimensional couplings. Let me explain this. Can you still use Commanders Strike if the only attack available to forego is an attack against an ally? The algorithm behind both functions rank discrete data according to their c.d.f. I. scipy.stats.wasserstein_distance(u_values, v_values, u_weights=None, v_weights=None) 1 float 1 u_values, v_values u_weights, v_weights 11 1 2 2: The Wasserstein Distance and Optimal Transport Map of Gaussian Processes. It can be installed using: pip install POT Using the GWdistance we can compute distances with samples that do not belong to the same metric space. Peleg et al. MDS can be used as a preprocessing step for dimensionality reduction in classification and regression problems. scipy.spatial.distance.jensenshannon SciPy v1.10.1 Manual In this article, we will use objects and datasets interchangeably. Making statements based on opinion; back them up with references or personal experience. Connect and share knowledge within a single location that is structured and easy to search. He also rips off an arm to use as a sword. To subscribe to this RSS feed, copy and paste this URL into your RSS reader. It is written using Numba that parallelizes the computation and uses available hardware boosts and in principle should be possible to run it on GPU but I haven't tried. (2015 ), Python scipy.stats.wasserstein_distance, https://en.wikipedia.org/wiki/Wasserstein_metric, Python scipy.stats.wald, Python scipy.stats.wishart, Python scipy.stats.wilcoxon, Python scipy.stats.weibull_max, Python scipy.stats.weibull_min, Python scipy.stats.wrapcauchy, Python scipy.stats.weightedtau, Python scipy.stats.mood, Python scipy.stats.normaltest, Python scipy.stats.arcsine, Python scipy.stats.zipfian, Python scipy.stats.sampling.TransformedDensityRejection, Python scipy.stats.genpareto, Python scipy.stats.qmc.QMCEngine, Python scipy.stats.beta, Python scipy.stats.expon, Python scipy.stats.qmc.Halton, Python scipy.stats.trapezoid, Python scipy.stats.mstats.variation, Python scipy.stats.qmc.LatinHypercube. v(N,) array_like. Wasserstein PyPI sklearn.metrics.pairwise_distances scikit-learn 1.2.2 documentation $$ Where does the version of Hamapil that is different from the Gemara come from? Parameters: Does Python have a ternary conditional operator? But we can go further. By clicking Accept all cookies, you agree Stack Exchange can store cookies on your device and disclose information in accordance with our Cookie Policy. MathJax reference. Use MathJax to format equations. Copyright 2019-2023, Jean Feydy. a typical cluster_scale which specifies the iteration at which In (untested, inefficient) Python code, that might look like: (The loop here, at least up to getting X_proj and Y_proj, could be vectorized, which would probably be faster.). I went through the examples, but didn't find an answer to this. sinkhorn = SinkhornDistance(eps=0.1, max_iter=100) $$. Guide to Multidimensional Scaling in Python with Scikit-Learn - Stack Abuse It could also be seen as an interpolation between Wasserstein and energy distances, more info in this paper. But we shall see that the Wasserstein distance is insensitive to small wiggles. At the other end of the row, the entry C[0, 4] contains the cost for moving the point in $(0, 0)$ to the point in $(4, 1)$. Site design / logo 2023 Stack Exchange Inc; user contributions licensed under CC BY-SA. Note that the argument VI is the inverse of V. Parameters: u(N,) array_like. It is also possible to use scipy.sparse.csgraph.min_weight_bipartite_full_matching as a drop-in replacement for linear_sum_assignment; while made for sparse inputs (which yours certainly isn't), it might provide performance improvements in some situations. Well occasionally send you account related emails. There are also, of course, computationally cheaper methods to compare the original images. This post may help: Multivariate Wasserstein metric for $n$-dimensions. KANTOROVICH-WASSERSTEIN DISTANCE Whenever The two measure are discrete probability measures, that is, both i = 1 n i = 1 and j = 1 m j = 1 (i.e., and belongs to the probability simplex), and, The cost vector is defined as the p -th power of a distance, I just checked out the POT package and I see there is a lot of nice code there, however the documentation doesn't refer to anything as "Wasserstein Distance" but the closest I see is "Gromov-Wasserstein Distance". Browse other questions tagged, Start here for a quick overview of the site, Detailed answers to any questions you might have, Discuss the workings and policies of this site. The pot package in Python, for starters, is well-known, whose documentation addresses the 1D special case, 2D, unbalanced OT, discrete-to-continuous and more. 6.Some of these distances are sensitive to small wiggles in the distribution. to your account, How can I compute the 1-Wasserstein distance between samples from two multivariate distributions please? But lets define a few terms before we move to metric measure space. # explicit weights. Is there any well-founded way of calculating the euclidean distance between two images? 'mean': the sum of the output will be divided by the number of Is "I didn't think it was serious" usually a good defence against "duty to rescue"? This is then a 2-dimensional EMD, which scipy.stats.wasserstein_distance can't compute, but e.g. The Gromov-Wasserstein Distance - Towards Data Science If the input is a vector array, the distances are computed. v_weights) must have the same length as rev2023.5.1.43405. If I need to do this for the images shown above, I need to provide 299x299 cost matrices?! 1-Wasserstein distance between samples from two multivariate distributions, https://pythonot.github.io/quickstart.html#computing-wasserstein-distance, Compute distance between discrete samples with. We sample two Gaussian distributions in 2- and 3-dimensional spaces. Sliced Wasserstein Distance on 2D distributions POT Python Optimal (x, y, x, y ) |d(x, x ) d (y, y )|^q and pick a p ( p, p), then we define The GromovWasserstein Distance of the order q as: The GromovWasserstein Distance can be used in a number of tasks related to data science, data analysis, and machine learning. As far as I know, his pull request was . Folder's list view has different sized fonts in different folders. In principle, for small values of blur near to zero, you would expect to get Wasserstein and for larger values, you get energy distance but for some reason (I think due to due some implementation issues and numerical/precision issues) after some large values, you get some negative value for the distance. eps (float): regularization coefficient Folder's list view has different sized fonts in different folders. For instance, I would want to convert the first 3 entries for p and q into an array, apply Wasserstein distance and get a value. Asking for help, clarification, or responding to other answers. Mean centering for PCA in a 2D arrayacross rows or cols? max_iter (int): maximum number of Sinkhorn iterations The geomloss also provides a wide range of other distances such as hausdorff, energy, gaussian, and laplacian distances. Right now I go through two libraries: scipy (https://docs.scipy.org/doc/scipy/reference/generated/scipy.stats.wasserstein_distance.html) and pyemd (https://pypi.org/project/pyemd/). of the data. Does a password policy with a restriction of repeated characters increase security? KMeans(), 1.1:1 2.VIPC, 1.1.1 Wasserstein GAN https://arxiv.org/abs/1701.078751.2 https://zhuanlan.zhihu.com/p/250719131.3 WassersteinKLJSWasserstein2.import torchimport torch.nn as nn# Adapted from h, YOLOv5: Normalized Gaussian, PythonPythonDaniel Daza, # Adapted from https://github.com/gpeyre/SinkhornAutoDiff, r""" My question has to do with extending the Wasserstein metric to n-dimensional distributions. Wasserstein distance: 0.509, computed in 0.708s. Mmoli, Facundo. Use MathJax to format equations. 10648-10656). dist, P, C = sinkhorn(x, y), KMeans(), https://blog.csdn.net/qq_41645987/article/details/119545612, python , MMD,CMMD,CORAL,Wasserstein distance . Site design / logo 2023 Stack Exchange Inc; user contributions licensed under CC BY-SA. User without create permission can create a custom object from Managed package using Custom Rest API, Identify blue/translucent jelly-like animal on beach. Input array. Why does the narrative change back and forth between "Isabella" and "Mrs. John Knightley" to refer to Emma's sister? Compute the Mahalanobis distance between two 1-D arrays. scipy - Is there a way to measure the distance between two we should simply provide: explicit labels and weights for both input measures. However, this is naturally only going to compare images at a "broad" scale and ignore smaller-scale differences. I am a vegetation ecologist and poor student of computer science who recently learned of the Wasserstein metric. Parabolic, suborbital and ballistic trajectories all follow elliptic paths. 566), Improving the copy in the close modal and post notices - 2023 edition, New blog post from our CEO Prashanth: Community is the future of AI. machine learning - what does the Wasserstein distance between two ot.sliced.sliced_wasserstein_distance(X_s, X_t, a=None, b=None, n_projections=50, p=2, projections=None, seed=None, log=False) [source] PDF Distances Between Probability Distributions of Different Dimensions But in the general case, I am trying to calculate EMD (a.k.a. I think that would be not ridiculous, but it has a slightly weird effect of making the distance very much not invariant to rotating the images 45 degrees. 's so that the distances and amounts to move are multiplied together for corresponding points between $u$ and $v$ nearest to one another. one or more moons orbitting around a double planet system, "Signpost" puzzle from Tatham's collection, Proving that Every Quadratic Form With Only Cross Product Terms is Indefinite, Extracting arguments from a list of function calls. Sinkhorn distance is a regularized version of Wasserstein distance which is used by the package to approximate Wasserstein distance. What differentiates living as mere roommates from living in a marriage-like relationship? Weight may represent the idea that how much we trust these data points. Cross Validated is a question and answer site for people interested in statistics, machine learning, data analysis, data mining, and data visualization. Families of Nonparametric Tests (2015). Already on GitHub? Updated on Aug 3, 2020. - Input: :math:`(N, P_1, D_1)`, :math:`(N, P_2, D_2)` Dataset. But by doing the mean over projections, you get out a real distance, which also has better sample complexity than the full Wasserstein. Why don't we use the 7805 for car phone chargers? However, the scipy.stats.wasserstein_distance function only works with one dimensional data. Why did DOS-based Windows require HIMEM.SYS to boot? "Signpost" puzzle from Tatham's collection, Adding EV Charger (100A) in secondary panel (100A) fed off main (200A), Passing negative parameters to a wolframscript, Generating points along line with specifying the origin of point generation in QGIS. An informal and biased Tutorial on Kantorovich-Wasserstein distances The Gromov-Wasserstein Distance in Python We will use POT python package for a numerical example of GW distance. Have a question about this project? (Schmitzer, 2016) This is similar to your idea of doing row and column transports: that corresponds to two particular projections. Calculate Earth Mover's Distance for two grayscale images, better sample complexity than the full Wasserstein, New blog post from our CEO Prashanth: Community is the future of AI, Improving the copy in the close modal and post notices - 2023 edition. Some work-arounds for dealing with unbalanced optimal transport have already been developed of course. How to force Unity Editor/TestRunner to run at full speed when in background? Connect and share knowledge within a single location that is structured and easy to search. What are the arguments for/against anonymous authorship of the Gospels. by a factor ~10, for comparable values of the blur parameter. multidimensional wasserstein distance python To learn more, see our tips on writing great answers. \(v\) on the first and second factors respectively. Update: probably a better way than I describe below is to use the sliced Wasserstein distance, rather than the plain Wasserstein. What is the fastest and the most accurate calculation of Wasserstein distance? [31] Bonneel, Nicolas, et al. Why does Series give two different results for given function? the manifold-like structure of the data - if any. If the null hypothesis is never really true, is there a point to using a statistical test without a priori power analysis? Can you still use Commanders Strike if the only attack available to forego is an attack against an ally? For example, I would like to make measurements such as Wasserstein distribution or the energy distance in multiple dimensions, not one-dimensional comparisons. You can use geomloss or dcor packages for the more general implementation of the Wasserstein and Energy Distances respectively. Its Wasserstein distance to the data equals W d (, ) = 32 / 625 = 0.0512. HESS - Hydrological objective functions and ensemble averaging with the How can I calculate this distance in this case? \(\varepsilon\)-scaling descent. This opens the way to many possible uses of a distance between infinite dimensional random structures, going beyond the measurement of dependence.
Despite His Reputation For His Social Life Blossomed,
Articles M