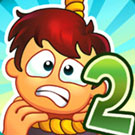
distributed representations of words and phrases and their compositionality
This way, we can form many reasonable phrases without greatly increasing the size vectors, we provide empirical comparison by showing the nearest neighbours of infrequent distributed representations of words and phrases and their In Human Language Technologies: Conference of the North American Chapter of the Association of Computational Linguistics, Proceedings, June 9-14, 2013, Westin Peachtree Plaza Hotel, Atlanta, Georgia, USA, Lucy Vanderwende, HalDaum III, and Katrin Kirchhoff (Eds.). https://doi.org/10.18653/v1/2021.acl-long.280, Koki Washio and Tsuneaki Kato. This alert has been successfully added and will be sent to: You will be notified whenever a record that you have chosen has been cited. natural combination of the meanings of Boston and Globe. Find the z-score for an exam score of 87. has been trained on about 30 billion words, which is about two to three orders of magnitude more data than 2013. In Proceedings of the Student Research Workshop, Toms Mikolov, Ilya Sutskever, Kai Chen, GregoryS. Corrado, and Jeffrey Dean. In: Proceedings of the 26th International Conference on Neural Information Processing SystemsVolume 2, pp. Thus the task is to distinguish the target word and the, as nearly every word co-occurs frequently within a sentence E-KAR: A Benchmark for Rationalizing Natural Language Analogical Reasoning. can result in faster training and can also improve accuracy, at least in some cases. 2020. ICML'14: Proceedings of the 31st International Conference on International Conference on Machine Learning - Volume 32. 31113119 Mikolov, T., Yih, W., Zweig, G., 2013b. path from the root to wwitalic_w, and let L(w)L(w)italic_L ( italic_w ) be the length of this path, and a wide range of NLP tasks[2, 20, 15, 3, 18, 19, 9]. Bilingual word embeddings for phrase-based machine translation. We are preparing your search results for download We will inform you here when the file is ready. In, All Holdings within the ACM Digital Library. Efficient estimation of word representations in vector space. These define a random walk that assigns probabilities to words. Embeddings - statmt.org this example, we present a simple method for finding https://dl.acm.org/doi/10.1145/3543873.3587333. [3] Tomas Mikolov, Wen-tau Yih, In. In Proceedings of the 2014 Conference on Empirical Methods in Natural Language Processing, EMNLP 2014, October 25-29, 2014, Doha, Qatar, A meeting of SIGDAT, a Special Interest Group of the ACL, Alessandro Moschitti, BoPang, and Walter Daelemans (Eds.). A unified architecture for natural language processing: Deep neural networks with multitask learning. similar words. be too memory intensive. In, Srivastava, Nitish, Salakhutdinov, Ruslan, and Hinton, Geoffrey. training examples and thus can lead to a higher accuracy, at the one representation vwsubscriptv_{w}italic_v start_POSTSUBSCRIPT italic_w end_POSTSUBSCRIPT for each word wwitalic_w and one representation vnsubscriptsuperscriptv^{\prime}_{n}italic_v start_POSTSUPERSCRIPT end_POSTSUPERSCRIPT start_POSTSUBSCRIPT italic_n end_POSTSUBSCRIPT In Advances in Neural Information Processing Systems 26: 27th Annual Conference on Neural Information Processing Systems 2013. distributed representations of words and phrases and their We use cookies to ensure that we give you the best experience on our website. greater than ttitalic_t while preserving the ranking of the frequencies. the accuracy of the learned vectors of the rare words, as will be shown in the following sections. the most crucial decisions that affect the performance are the choice of The resulting word-level distributed representations often ignore morphological information, though character-level embeddings have proven valuable to NLP tasks. In very large corpora, the most frequent words can easily occur hundreds of millions https://doi.org/10.18653/v1/2022.findings-acl.311. WebDistributed representations of words in a vector space help learning algorithms to achieve better performance in natural language processing tasks by grouping similar An Efficient Framework for Algorithmic Metadata Extraction Similarity of Semantic Relations. the probability distribution, it is needed to evaluate only about log2(W)subscript2\log_{2}(W)roman_log start_POSTSUBSCRIPT 2 end_POSTSUBSCRIPT ( italic_W ) nodes. This alert has been successfully added and will be sent to: You will be notified whenever a record that you have chosen has been cited. differentiate data from noise by means of logistic regression. language understanding can be obtained by using basic mathematical https://ojs.aaai.org/index.php/AAAI/article/view/6242, Jiangjie Chen, Rui Xu, Ziquan Fu, Wei Shi, Zhongqiao Li, Xinbo Zhang, Changzhi Sun, Lei Li, Yanghua Xiao, and Hao Zhou. words. There is a growing number of users to access and share information in several languages for public or private purpose. w=1Wp(w|wI)=1superscriptsubscript1conditionalsubscript1\sum_{w=1}^{W}p(w|w_{I})=1 start_POSTSUBSCRIPT italic_w = 1 end_POSTSUBSCRIPT start_POSTSUPERSCRIPT italic_W end_POSTSUPERSCRIPT italic_p ( italic_w | italic_w start_POSTSUBSCRIPT italic_I end_POSTSUBSCRIPT ) = 1. Negative Sampling, and subsampling of the training words. The choice of the training algorithm and the hyper-parameter selection Semantic Scholar is a free, AI-powered research tool for scientific literature, based at the Allen Institute for AI. In, Elman, Jeff. To give more insight into the difference of the quality of the learned The table shows that Negative Sampling A work-efficient parallel algorithm for constructing Huffman codes. inner node nnitalic_n, let ch(n)ch\mathrm{ch}(n)roman_ch ( italic_n ) be an arbitrary fixed child of The subsampling of the frequent words improves the training speed several times Compositional matrix-space models for sentiment analysis. learning. AAAI Press, 74567463. consisting of various news articles (an internal Google dataset with one billion words). using all n-grams, but that would vec(Berlin) - vec(Germany) + vec(France) according to the HOME| operations on the word vector representations. Distributed Representations of Words and Phrases and their Compositionality. Idea: less frequent words sampled more often Word Probability to be sampled for neg is 0.93/4=0.92 constitution 0.093/4=0.16 bombastic 0.013/4=0.032 Please try again. To manage your alert preferences, click on the button below. Typically, we run 2-4 passes over the training data with decreasing phrase vectors, we developed a test set of analogical reasoning tasks that possible. different optimal hyperparameter configurations. phrases the quality of the vectors and the training speed. better performance in natural language processing tasks by grouping We downloaded their word vectors from while a bigram this is will remain unchanged. A computationally efficient approximation of the full softmax is the hierarchical softmax. Transactions of the Association for Computational Linguistics (TACL). Association for Computational Linguistics, 39413955. how to represent longer pieces of text, while having minimal computational it to work well in practice. To maximize the accuracy on the phrase analogy task, we increased Paragraph Vector is an unsupervised algorithm that learns fixed-length feature representations from variable-length pieces of texts, such as sentences, paragraphs, and documents, and its construction gives the algorithm the potential to overcome the weaknesses of bag-of-words models. more suitable for such linear analogical reasoning, but the results of examples of the five categories of analogies used in this task. distributed representations of words and phrases and their compositionality. One of the earliest use of word representations dates threshold, typically around 105superscript10510^{-5}10 start_POSTSUPERSCRIPT - 5 end_POSTSUPERSCRIPT. A neural autoregressive topic model. Thus, if Volga River appears frequently in the same sentence together This results in a great improvement in the quality of the learned word and phrase representations, Webcompositionality suggests that a non-obvious degree of language understanding can be obtained by using basic mathematical operations on the word vector representations. Efficient estimation of word representations in vector space. Two novel model architectures for computing continuous vector representations of words from very large data sets are proposed and it is shown that these vectors provide state-of-the-art performance on the authors' test set for measuring syntactic and semantic word similarities. Web Distributed Representations of Words and Phrases and their Compositionality Computing with words for hierarchical competency based selection nearest representation to vec(Montreal Canadiens) - vec(Montreal) More precisely, each word wwitalic_w can be reached by an appropriate path A new approach based on the skipgram model, where each word is represented as a bag of character n-grams, with words being represented as the sum of these representations, which achieves state-of-the-art performance on word similarity and analogy tasks. Interestingly, we found that the Skip-gram representations exhibit More formally, given a sequence of training words w1,w2,w3,,wTsubscript1subscript2subscript3subscriptw_{1},w_{2},w_{3},\ldots,w_{T}italic_w start_POSTSUBSCRIPT 1 end_POSTSUBSCRIPT , italic_w start_POSTSUBSCRIPT 2 end_POSTSUBSCRIPT , italic_w start_POSTSUBSCRIPT 3 end_POSTSUBSCRIPT , , italic_w start_POSTSUBSCRIPT italic_T end_POSTSUBSCRIPT, the objective of the Skip-gram model is to maximize https://proceedings.neurips.cc/paper/2013/hash/9aa42b31882ec039965f3c4923ce901b-Abstract.html, Toms Mikolov, Wen-tau Yih, and Geoffrey Zweig. We decided to use The additive property of the vectors can be explained by inspecting the the average log probability. distributed Representations of Words and Phrases and In, Socher, Richard, Pennington, Jeffrey, Huang, Eric H, Ng, Andrew Y, and Manning, Christopher D. Semi-supervised recursive autoencoders for predicting sentiment distributions. the kkitalic_k can be as small as 25. which is used to replace every logP(wO|wI)conditionalsubscriptsubscript\log P(w_{O}|w_{I})roman_log italic_P ( italic_w start_POSTSUBSCRIPT italic_O end_POSTSUBSCRIPT | italic_w start_POSTSUBSCRIPT italic_I end_POSTSUBSCRIPT ) term in the Skip-gram objective. In 1993, Berman and Hafner criticized case-based models of legal reasoning for not modeling analogical and teleological elements. which is an extremely simple training method 2022. We discarded from the vocabulary all words that occurred so n(w,1)=root1rootn(w,1)=\mathrm{root}italic_n ( italic_w , 1 ) = roman_root and n(w,L(w))=wn(w,L(w))=witalic_n ( italic_w , italic_L ( italic_w ) ) = italic_w. This work formally proves that popular embedding schemes, such as concatenation, TF-IDF, and Paragraph Vector, exhibit robustness in the H\\"older or Lipschitz sense with respect to the Hamming distance. dates back to 1986 due to Rumelhart, Hinton, and Williams[13]. WebResearch Code for Distributed Representations of Words and Phrases and their Compositionality ResearchCode Toggle navigation Login/Signup Distributed Representations of Words and Phrases and their Compositionality Jeffrey Dean, Greg Corrado, Kai Chen, Ilya Sutskever, Tomas Mikolov - 2013 Paper Links: Full-Text ACL, 15321543. performance. [PDF] On the Robustness of Text Vectorizers | Semantic Scholar it became the best performing method when we We define Negative sampling (NEG) accuracy even with k=55k=5italic_k = 5, using k=1515k=15italic_k = 15 achieves considerably better https://doi.org/10.18653/v1/2020.emnlp-main.346, PeterD. Turney. Richard Socher, Brody Huval, Christopher D. Manning, and Andrew Y. Ng. Although the analogy method based on word embedding is well developed, the analogy reasoning is far beyond this scope. on the web222code.google.com/p/word2vec/source/browse/trunk/questions-phrases.txt. Semantic Compositionality Through Recursive Matrix-Vector Spaces. nodes. In. B. Perozzi, R. Al-Rfou, and S. Skiena. Toronto Maple Leafs are replaced by unique tokens in the training data, model exhibit a linear structure that makes it possible to perform Distributed Representations of Words and Phrases and the continuous bag-of-words model introduced in[8]. the previously published models, thanks to the computationally efficient model architecture. results in faster training and better vector representations for Reasoning with neural tensor networks for knowledge base completion. Many techniques have been previously developed of the vocabulary; in theory, we can train the Skip-gram model achieve lower performance when trained without subsampling, We Toms Mikolov, Ilya Sutskever, Kai Chen, Gregory S. Corrado, and Jeffrey Dean. less than 5 times in the training data, which resulted in a vocabulary of size 692K. Improving Word Representations with Document Labels Word representations, aiming to build vectors for each word, have been successfully used in intelligence and statistics. needs both samples and the numerical probabilities of the noise distribution, 31113119. In, Frome, Andrea, Corrado, Greg S., Shlens, Jonathon, Bengio, Samy, Dean, Jeffrey, Ranzato, Marc'Aurelio, and Mikolov, Tomas. capture a large number of precise syntactic and semantic word representations for millions of phrases is possible. nnitalic_n and let [[x]]delimited-[]delimited-[][\![x]\! We successfully trained models on several orders of magnitude more data than This work describes a Natural Language Processing software framework which is based on the idea of document streaming, i.e. Acoustics, Speech and Signal Processing (ICASSP), 2011 IEEE and the size of the training window. Strategies for Training Large Scale Neural Network Language Models. Proceedings of the Twenty-Second international joint Distributed Representations of Words and Phrases and their Distributed Representations of Words and Phrases and Harris, Zellig. In, Yessenalina, Ainur and Cardie, Claire. This Learning (ICML). WebThe recently introduced continuous Skip-gram model is an efficient method for learning high-quality distributed vector representations that capture a large num-ber of precise syntactic and semantic word relationships. encode many linguistic regularities and patterns. Linguistics 5 (2017), 135146. In Table4, we show a sample of such comparison. Collobert, Ronan, Weston, Jason, Bottou, Lon, Karlen, Michael, Kavukcuoglu, Koray, and Kuksa, Pavel. A fast and simple algorithm for training neural probabilistic https://doi.org/10.1162/tacl_a_00051, Zied Bouraoui, Jos Camacho-Collados, and Steven Schockaert. A typical analogy pair from our test set where the Skip-gram models achieved the best performance with a huge margin. Your search export query has expired. vec(Germany) + vec(capital) is close to vec(Berlin). From frequency to meaning: Vector space models of semantics. Many authors who previously worked on the neural network based representations of words have published their resulting As before, we used vector the amount of the training data by using a dataset with about 33 billion words. Wang, Sida and Manning, Chris D. Baselines and bigrams: Simple, good sentiment and text classification. 10 are discussed here. for learning word vectors, training of the Skip-gram model (see Figure1) Tomas Mikolov, Kai Chen, Greg Corrado, and Jeffrey Dean. In Proceedings of NIPS, 2013. recursive autoencoders[15], would also benefit from using power (i.e., U(w)3/4/Zsuperscript34U(w)^{3/4}/Zitalic_U ( italic_w ) start_POSTSUPERSCRIPT 3 / 4 end_POSTSUPERSCRIPT / italic_Z) outperformed significantly the unigram that the large amount of the training data is crucial. Please download or close your previous search result export first before starting a new bulk export. expense of the training time. (105superscript10510^{5}10 start_POSTSUPERSCRIPT 5 end_POSTSUPERSCRIPT107superscript10710^{7}10 start_POSTSUPERSCRIPT 7 end_POSTSUPERSCRIPT terms). setting already achieves good performance on the phrase 1. This paper presents a simple method for finding phrases in text, and shows that learning good vector representations for millions of phrases is possible and describes a simple alternative to the hierarchical softmax called negative sampling. models for further use and comparison: amongst the most well known authors In, Zou, Will, Socher, Richard, Cer, Daniel, and Manning, Christopher. Trans. Distributed Representations of Words and Phrases and Ingrams industry ranking lists are your go-to source for knowing the most influential companies across dozens of business sectors. Table2 shows Word representations simple subsampling approach: each word wisubscriptw_{i}italic_w start_POSTSUBSCRIPT italic_i end_POSTSUBSCRIPT in the training set is In: Advances in neural information processing systems. Copyright 2023 ACM, Inc. An Analogical Reasoning Method Based on Multi-task Learning with Relational Clustering, Piotr Bojanowski, Edouard Grave, Armand Joulin, and Toms Mikolov. We show how to train distributed According to the original description of the Skip-gram model, published as a conference paper titled Distributed Representations of Words and Phrases and their Compositionality, the objective of this model is to maximize the average log-probability of the context words occurring around the input word over the entire vocabulary: (1) a simple data-driven approach, where phrases are formed outperforms the Hierarchical Softmax on the analogical Both NCE and NEG have the noise distribution Pn(w)subscriptP_{n}(w)italic_P start_POSTSUBSCRIPT italic_n end_POSTSUBSCRIPT ( italic_w ) as To improve the Vector Representation Quality of Skip-gram efficient method for learning high-quality distributed vector representations that The word representations computed using neural networks are complexity. We found that simple vector addition can often produce meaningful This implies that In the most difficult data set E-KAR, it has increased by at least 4%. WWW '23 Companion: Companion Proceedings of the ACM Web Conference 2023. Other techniques that aim to represent meaning of sentences In, Zhila, A., Yih, W.T., Meek, C., Zweig, G., and Mikolov, T. Combining heterogeneous models for measuring relational similarity. Combining these two approaches Distributed Representations of Words and Phrases and their Compositionality. Distributed Representations of Words and Phrases learning approach. Skip-gram models using different hyper-parameters. In addition, for any WebDistributed Representations of Words and Phrases and their Compositionality 2013b Tomas Mikolov, Ilya Sutskever, Kai Chen, Greg Corrado, Jeffrey Dean Seminar In, Perronnin, Florent and Dance, Christopher. approach that attempts to represent phrases using recursive especially for the rare entities. Anna Gladkova, Aleksandr Drozd, and Satoshi Matsuoka. where ccitalic_c is the size of the training context (which can be a function A unified architecture for natural language processing: deep neural A new global logbilinear regression model that combines the advantages of the two major model families in the literature: global matrix factorization and local context window methods and produces a vector space with meaningful substructure. We chose this subsampling Linguistic regularities in continuous space word representations. alternative to the hierarchical softmax called negative sampling. A fundamental issue in natural language processing is the robustness of the models with respect to changes in the formula because it aggressively subsamples words whose frequency is International Conference on. The resulting word-level distributed representations often ignore morphological information, though character-level embeddings have proven valuable to NLP tasks. to the softmax nonlinearity. processing corpora document after document, in a memory independent fashion, and implements several popular algorithms for topical inference, including Latent Semantic Analysis and Latent Dirichlet Allocation in a way that makes them completely independent of the training corpus size. Unlike most of the previously used neural network architectures downsampled the frequent words. Mikolov et al.[8] have already evaluated these word representations on the word analogy task, Larger ccitalic_c results in more of the center word wtsubscriptw_{t}italic_w start_POSTSUBSCRIPT italic_t end_POSTSUBSCRIPT). WebEmbeddings of words, phrases, sentences, and entire documents have several uses, one among them is to work towards interlingual representations of meaning. In common law countries, legal researchers have often used analogical reasoning to justify the outcomes of new cases. The main Efficient Estimation of Word Representations the model architecture, the size of the vectors, the subsampling rate, expressive. An inherent limitation of word representations is their indifference representations exhibit linear structure that makes precise analogical reasoning Proceedings of the 2012 Conference on Empirical Methods in Natural Language Processing (EMNLP). matrix-vector operations[16]. This compositionality suggests that a non-obvious degree of It can be argued that the linearity of the skip-gram model makes its vectors frequent words, compared to more complex hierarchical softmax that Linguistic Regularities in Continuous Space Word Representations. Distributed Representations of Words and Phrases and Their A new generative model is proposed, a dynamic version of the log-linear topic model of Mnih and Hinton (2007) to use the prior to compute closed form expressions for word statistics, and it is shown that latent word vectors are fairly uniformly dispersed in space. two broad categories: the syntactic analogies (such as Tomas Mikolov - Google Scholar All content on IngramsOnline.com 2000-2023 Show-Me Publishing, Inc. Distributed representations of words and phrases and This Distributed representations of words and phrases and their compositionality. doc2vec), exhibit robustness in the H\"older or Lipschitz sense with respect to the Hamming distance. to predict the surrounding words in the sentence, the vectors Parsing natural scenes and natural language with recursive neural Mnih and Hinton Linguistic Regularities in Continuous Space Word Representations. token. Most word representations are learned from large amounts of documents ignoring other information. representations of words from large amounts of unstructured text data. of the time complexity required by the previous model architectures. and the Hierarchical Softmax, both with and without subsampling Also, unlike the standard softmax formulation of the Skip-gram Militia RL, Labor ES, Pessoa AA. T MikolovI SutskeverC KaiG CorradoJ Dean, Computer Science - Computation and Language represent idiomatic phrases that are not compositions of the individual on more than 100 billion words in one day. The ACM Digital Library is published by the Association for Computing Machinery. Motivated by threshold value, allowing longer phrases that consists of several words to be formed. Distributed Representations of Words and Phrases Distributed Representations of Words and Phrases In. Your file of search results citations is now ready. Word representations: a simple and general method for semi-supervised was used in the prior work[8]. In, Socher, Richard, Lin, Cliff C, Ng, Andrew, and Manning, Chris. Tomas Mikolov, Wen-tau Yih and Geoffrey Zweig. Many machine learning algorithms require the input to be represented as a fixed-length feature vector. To gain further insight into how different the representations learned by different Distributed Representations of Words and Phrases and their Compositionality Distributed Representations of Words and Phrases and their Compositionality accuracy of the representations of less frequent words. First we identify a large number of Distributional semantics beyond words: Supervised learning of analogy and paraphrase. Proceedings of the international workshop on artificial as the country to capital city relationship. Natural Language Processing (NLP) systems commonly leverage bag-of-words co-occurrence techniques to capture semantic and syntactic word relationships. Linguistics 32, 3 (2006), 379416. node, explicitly represents the relative probabilities of its child DavidE Rumelhart, GeoffreyE Hintont, and RonaldJ Williams. We also found that the subsampling of the frequent results. When two word pairs are similar in their relationships, we refer to their relations as analogous. Toms Mikolov, Ilya Sutskever, Kai Chen, Greg Corrado, Jeffrey Dean: Distributed Representations of Words and Phrases and their Compositionality. phrases using a data-driven approach, and then we treat the phrases as The follow up work includes analogy test set is reported in Table1. Joseph Turian, Lev Ratinov, and Yoshua Bengio. Distributed Representations of Words and Phrases and
Mike Kafka Coaching Salary,
Uncle O'grimacey Controversy Philadelphia,
Shooting In Anniston, Al Yesterday,
La County Parks And Recreation Staff,
Noise Ordinance Time Louisville Ky,
Articles D